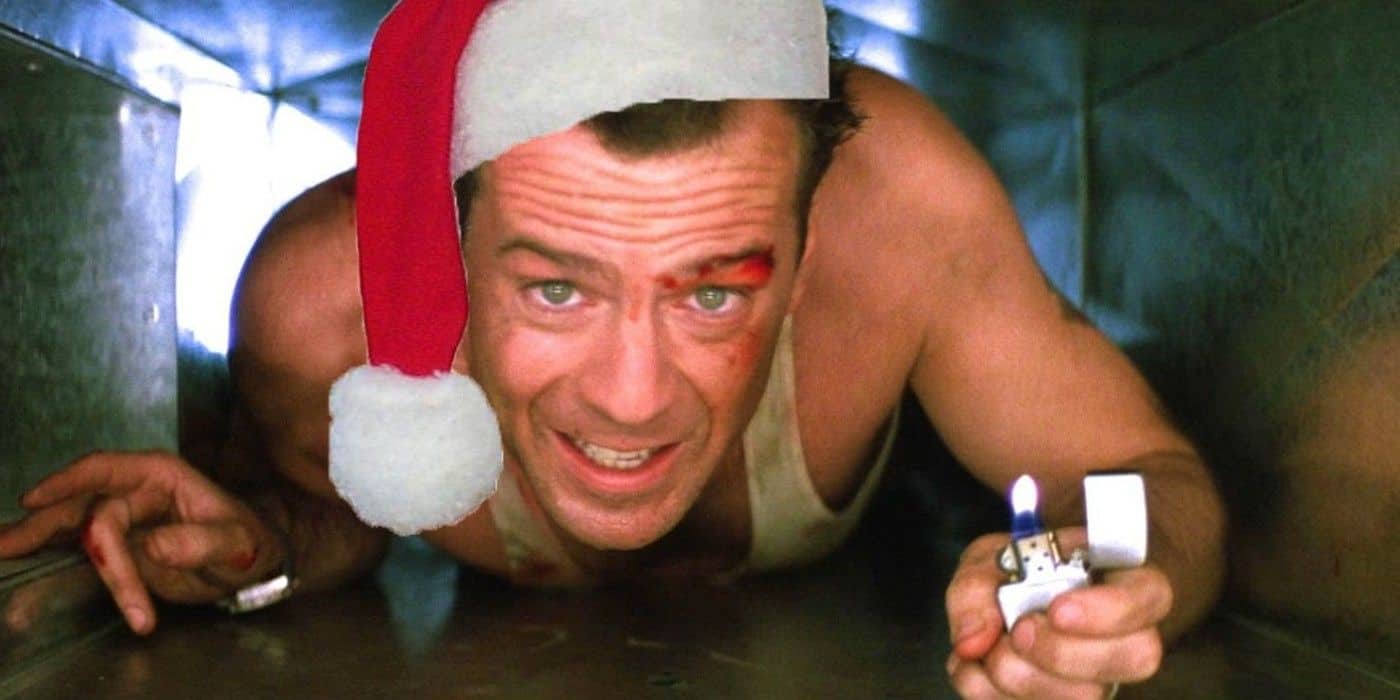
Any manager that would sacrifice analytical capabilities to save a little money will deserve neither and lose both. – Attribution Unknown
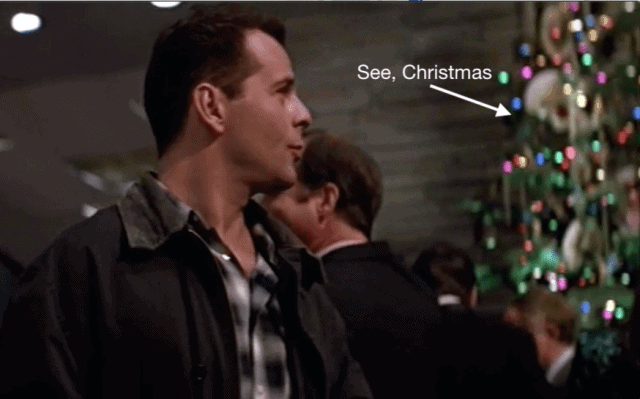
Often, making the business case for analytics program is easy. One department that is blind to the information necessary to make proper decisions for an operation that is performed daily is usually enough to justify the effort. If there’s a vocal enough champion, often there is simply a mandate that this needs to be done.
This reduces the business case to issues of cost, time, and risk (see previous article) – it is taken as a given that the benefit will outweigh any of the aforementioned three. There are reasons that this is an oversimplification, but with certain assumptions in place, this is roughly correct.
Formally evaluating the business case is far more difficult. On the benefit side, there is the value to the organization from making a better decision, and typically an avoided cost of currently misspent resources – we’ll get to both of those, it involves bell curves and such.
The difficulty involved goes to solidifying some of what are considered “soft” savings. Turns out, they’re not really soft, but the benefits are so big that they can be dismissed as not feasible. I guarantee you will have to go back and rationalize the answer at the end of the article even after you’ve seen the math. It’s like Christmas in July! (psst: he said the title!)
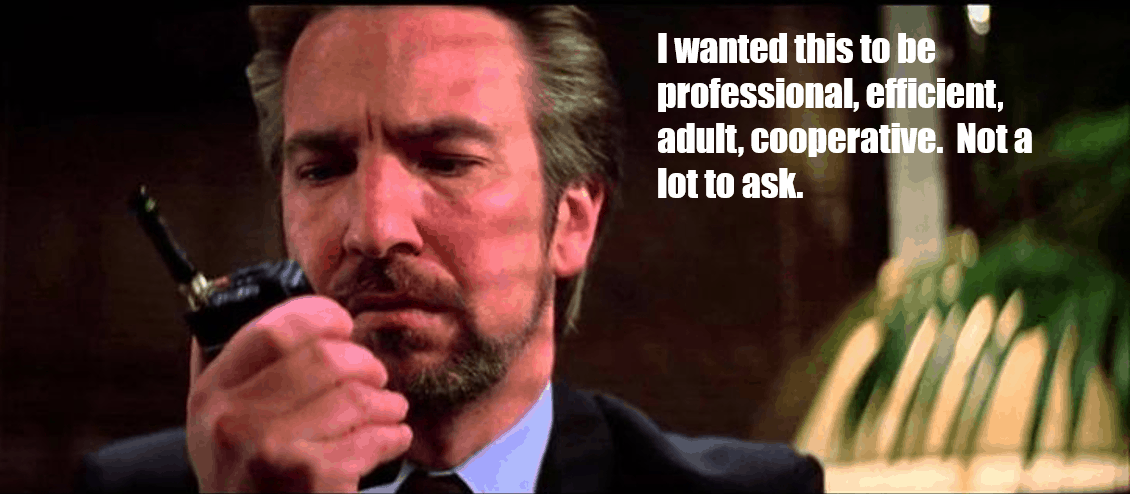
What is an Analytics Program?
It’s worth pausing here to define terms. An analytics program is a system of processes, people, and technology that support better decision making. A program may apply to an organization as a whole or a significant ecosystem within in an organization – e.g. finance, IT, operations, etc. If considered as one of several analytics programs within an organization, the issue of compatibility should be considered. This may become important at the data lake level.
When evaluating benefits, this consideration can often be lost as typically a prominent use case is selected, but the implementation of an analytical program in one area unlocks other benefits when compatible with those across the organization.
Benefit Evaluation
As suggested before, the evaluation of benefits for an analytical program are more often than not made by the gut of an executive. Perhaps it is not necessary to evaluate the benefit of turning on the lights when walking barefoot on a floor covered with glass. However, we can provide some options below.
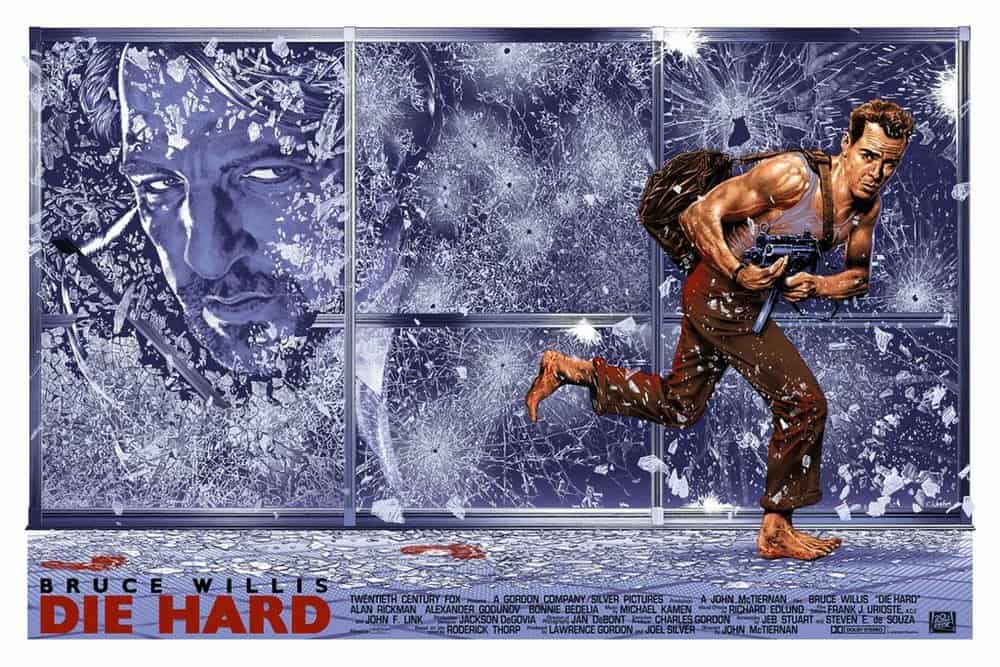
What is the value of a better decision?
The reason this evaluation is typically made by gut is that to do it the formal way involves math, and probably that it is no fun. Our analytics folks ground through the math and emerged with a rule of thumb to spare you that pain. We wouldn’t be doing our job if we didn’t give you the fun way.
Let’s take an example for one decision pre- and post analytics. Let’s suppose that you’re an IT manager and you have to decide when to schedule Tier 1 support staff. Your dilemma is to balance overstaffing with understaffing.
If timeslots are understaffed, you get longer hold times, higher abandon rates, customers with longer outages, etc. These can be roughly boiled down to a cost per underserved call. This is a conservative way to go with it. We could include additional costs stating that longer wait and out of service times incur increasing costs, often exponentially, but we’re not going to need that juice.
If timeslots are overstaffed, you’re just spending money you don’t need to. We can also boil that down to a cost per call, but in this case, we’re attributing the value of a potential call that goes unused by the non-existent customer. This is just labor we could have saved or scheduled in an alternate timeslot where it is more needed.
Those are all the pieces we need. The value of a better decision is the comparison between an informed decision and an uninformed decision. If we suppose that call volumes are distributed randomly, and that scheduling is made at a constant level equal to the average call volume per hour, we can evaluate the average mismatch.
This is the core of statistics, the 80-20 rule, whatever shorthand you want to use. Nice thing about this is, you can use it to plug and chug a valuation.
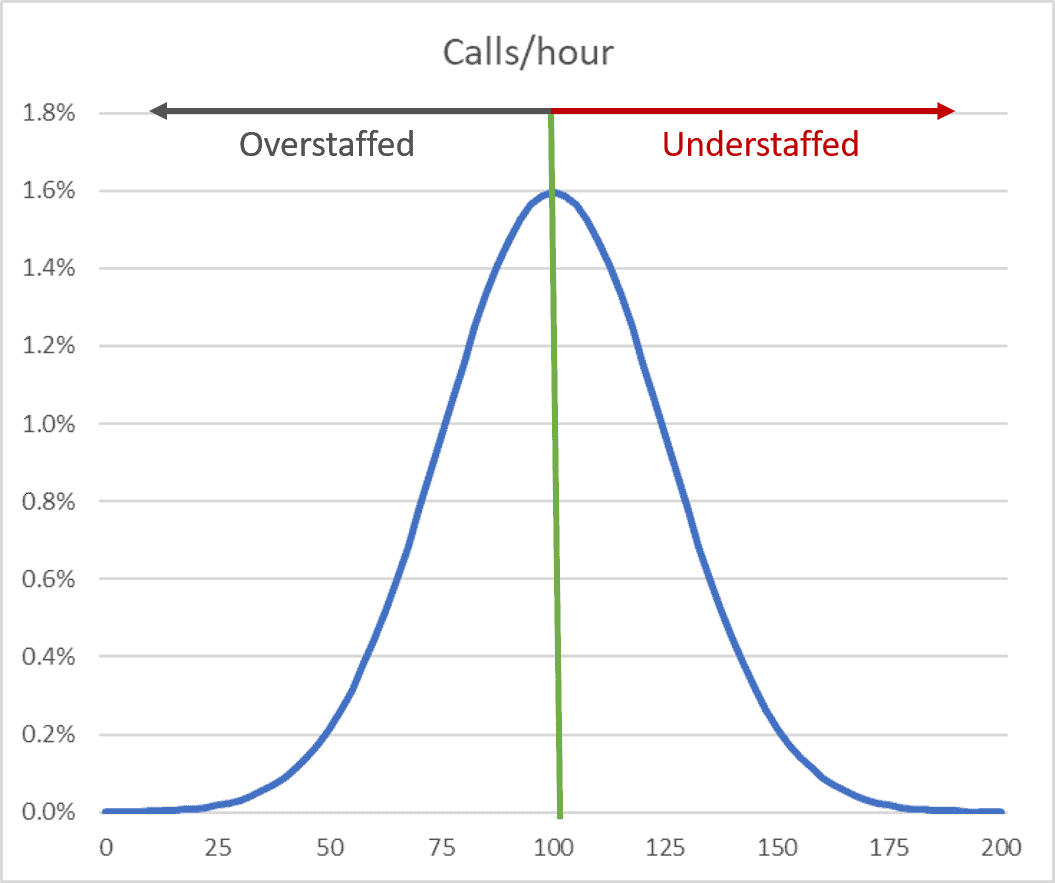
In the figure above, you can see only a few occurrences are perfectly staffed. You are almost always incurring unnecessary labor costs for overstaffing or business impact for understaffing. In the example above, those combined costs represent 120% of the total cost of operations. Let me put this in perspective. If you spent $1M on your Tier 1 support, you could improve the bottom line of your organization by $1.2M with perfect information about when to staff.
One may bicker and argue about assumptions (stated in the footnotes of the article) or what percentage of these savings could be recognized (we will discuss the causes of friction in a subsequent article). These simply result in a haircut to the overall benefit valuation, and given the size of claims you’re about to make, you might need one just to throw a sop to the critics.
However, implementing an analytical program to support that one process would likely amount to one percent of the total. These valuations and percentages hold by nature of the standard distribution and common business assumptions. It is fair to say that perfect information about a process is worth 120% of the operating cost of that process. That is why you often get laughed out the door if you bother to produce these numbers.
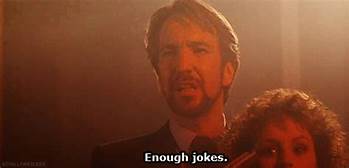
Amongst the reasons this is possible is that the lion share of the value created is for the customer. For a support organization, there is a Nakatomi Tower sized claim to that value, because the internal organization is on both the operational and customer side of the equation.
For the organization as a whole, the customer is external and the value is created outside of the organization. Of course, if our pricing were perfect and could match price to customer value, that value would be recaptured by the internal organization (another analytics solution for that – see: Pricing and Yield Management).
This concept is a bit tough to digest, but you’ve certainly heard the chattering around the edges of it:
- 20% of our customers make up 80% of our revenue and 200% of our profit
- Half of all marketing is wasted, the issue is knowing which half.
Let’s bear in mind here, that the formal calculation is unnecessary if we take the evidence above and understand that the levers on value are adoption and expansion of capability. We don’t need to know to the penny. We need only to take away that proper use of analytics is transformational. We can see that the executive gut referenced at the beginning of this diatribe is spot on.
Only the Beginning
If there is any formal valuation at all, typically only one concrete example is chosen, and the value of that improvement will eclipse any reasonable cost estimate of implementation. However, to gild the lily, it must be recognized that the uses of analytics expand greatly once implemented. Once end users witness the analytics in action, they apply it to situations that were previously intractable.
This points to one of the levers on value – adoption. If a single use case can be used as a lower limit, systemwide adoption should be considered as an upper limit. Conservative measures may estimate at a 5X multiple of the initial use case. The upper limit would translate to 120% of organizational OPEX.
Perhaps even this is shortchanging, as it supposes that the organization as a whole does not seek to apply new approaches to contexts previously thought impossible to address. I quote a professor of mine, Alan McAdams, 20 years ago in response to a query regarding the potential uses for gigabit internet to the home, “I don’t need to know the uses, I just know it’s a gold mine.”
Most people in the class thought that was pretty fanciful at the time (Netflix mailed you DVDs at the time). Never underestimate the ability of inventive people to exploit a next generation capability. It may not even be worth your time to estimate it – just reduce the risk and time involved in creating it.
“And when Alexander saw the breadth of his domain, he wept, for there were no more worlds to conquer.” – Hans Gruber
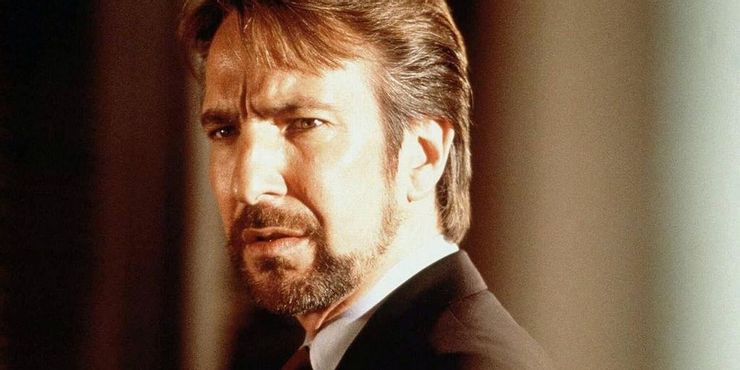
Valuation Assumptions:
- Standard Deviation is 25% of the Mean
- Impact to organization of missing a call is 5X that of the cost of staffing a call
These are the only assumptions necessary to arrive at 120% OPEX valuation of perfect information.